Computational Models from Medical Images
Cerebrovascular Modeling: MRI Angio-/Venography Vectorization and Flow Simulation Heading link
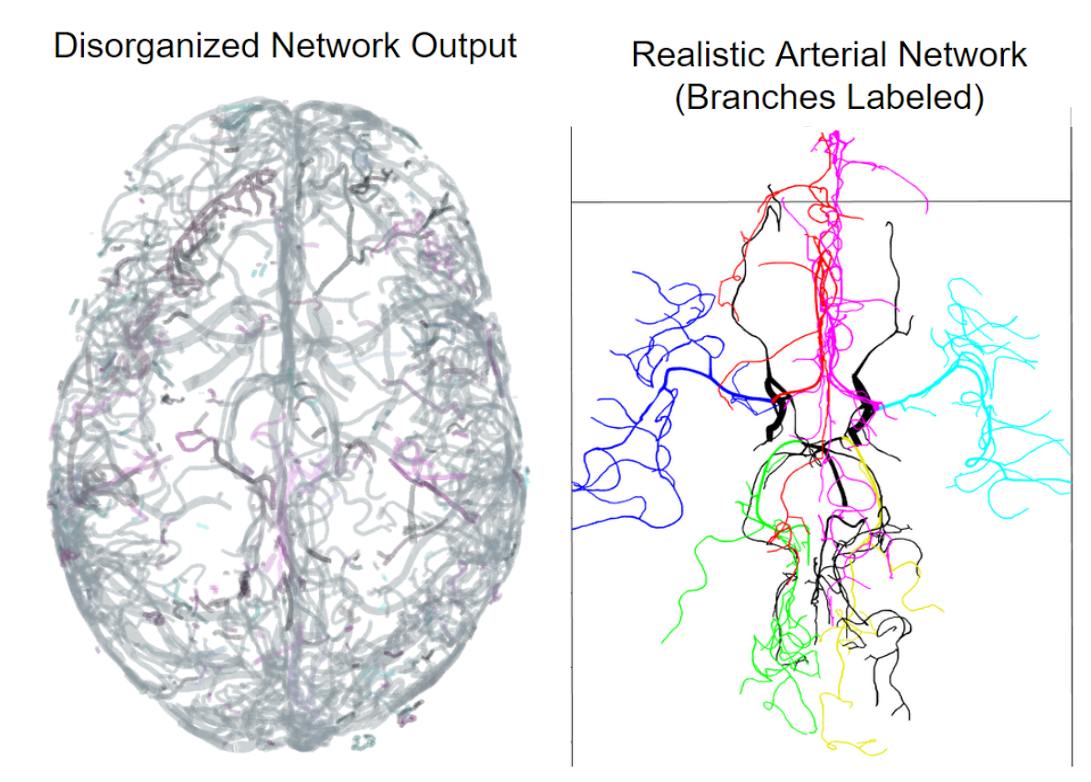
Cerebrovascular Modeling: MRI Angio-/Venography Vectorization and Flow Simulation
Samuel Mihelic, Roxana Dupuy, Lena Bouvier, Andreas Linninger
The goal of this project is to extract a more complete, accurate, and realistic model of the human cerebrovasculature from magnetic resonance angiography (MRA) and venography (MRV) images. Bitmap representations of the vascular network are vectorized using the state-of-the-art Segmentation-Less Automated Vascular Vectorization (SLAVV) method, which is optimized for accurate vessel sizing, but not for fluid-flow simulation. The resulting disorganized, disconnected set of vectors is then post-processed into a single, connected component, that can be used in a one-dimensional blood flow simulation. In the case of the MRV, the high-pressure arterial inlets are identified, and a “ground” pressure is assigned at the outlets where the arterial tree becomes too small to resolve (<1 mm diameter). The fluid flow simulation is used to orient the vessel branches and identify bifurcations and unions at junction points. Erroneous venous components are removed using results from the blood flow simulation, resulting in a more realistic model of the network. This computational pipeline was applied to six subjects (whose concise vectorized representation will be made publicly available), and is being used to check hypotheses related to Murray’s Law and Strahler Order.
Computational Models From Medical Images Heading link
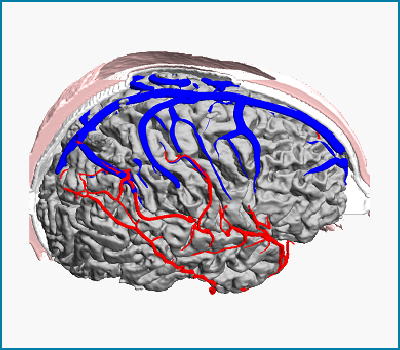
Whole Brain Computational Mesh Generation
Chih-Yang Hsu, Ben Schneller and Andreas Linninger
Subject-specific anatomical data is acquired by medical imaging modalities such as magnetic resonance imaging (MRI), computed tomography (CT) or digital subtraction angiography (DSA) to delineate the main geometric features of individual patients’ brains. We develop an imaging pipeline based on automatic image reconstruction algorithms for creating computational meshes for the human brain that extract detailed anatomical structures such as the arterial and venous trees, the skull and scalp, as well as the cortical surface. Anatomically detailed models enable subject-specific computational fluid dynamic simulations of the cerebral blood flow or highly realistic visualizations of the human brain.
Parametric Mesh Generation for Cerebral Vasculature Heading link
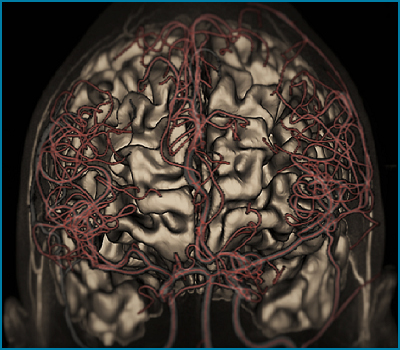
Parametric Mesh Generation for Cerebral Vasculature
Mahsa Ghaffari and Andreas Linninger
Computational meshes of the cerebral angioarchitecture are a prerequisite for the study of blood flow patterns in the entire brain. Because existing computational meshing techniques require massive numbers of elements, new algorithms are being developed for smooth vessel network reconstruction to enable fast and more precise computational fluid dynamic simulations. A novel fully-automatic algorithm for structured parametric meshing is created to reconstruct the anatomy of blood flow networks from medical images without user supervision. Subject-specific representations of vascular trees are necessary to perform organ-wide hemodynamics for personalized surgical planning of vascular disease interventions.
Algorithms for Reconstruction of Cerebral Angioarchitecture from Medical Images Heading link
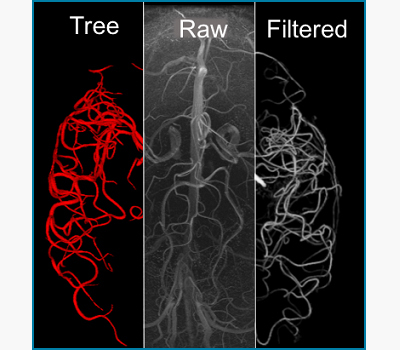
Algorithms for Reconstruction of Cerebral Angioarchitecture from Medical Images
Ben Schneller, Chih-Yang Hsu, Mahsa Ghaffari and Andreas Linninger
We develop novel image filter algorithms to enhance the contrast from tortuous blood vessels acquired by magnetic resonance angiography (MRA) and venography (MRV), while suppressing the signal from the cortex and imaging noise. Filtered images facilitate the automatic segmentation of subject-specific arterial or venous tree. The reconstructed networks can be used for scientific visualization of brain data, training of medical students,automatic mesh generation for CFD simulations as well as blueprints for realistic blood flow networks fabricated with 3d printing technology.